AI for agricultural innovation is a game-changer that has the potential to revolutionize the farming industry.
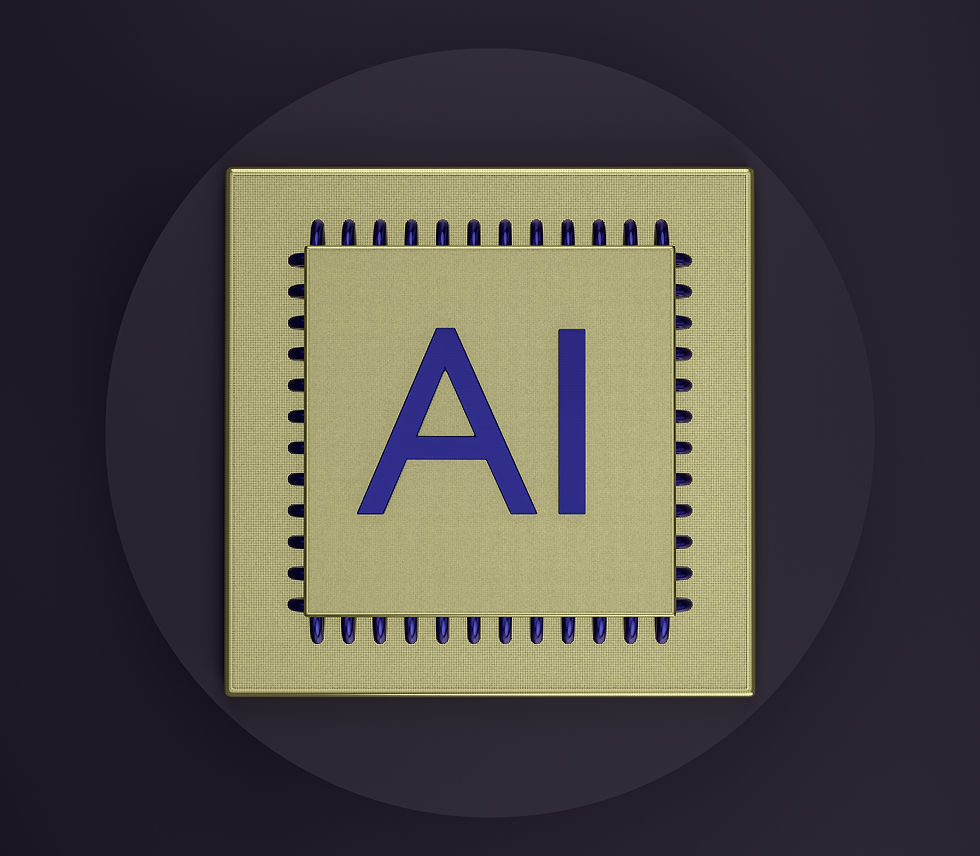
With the help of AI technology, farmers can gather and analyze vast amounts of data to make informed decisions about crop management, optimizing resources, and improving yields.
Additionally, AI can assist in predicting weather patterns and disease outbreaks, enabling farmers to take proactive measures to protect their crops ( Ennouri et al., 2021).
Furthermore, AI and machine learning can be utilized for precision agriculture, where real-time sensor data from drones and visual analytics are used to improve crop yield predictions and optimize the use of resources such as water and fertilizers (Liu et al., 2022).
Overall, AI for agricultural innovation holds the promise of improving efficiency, profitability, and sustainability in farming practices.
The vision of AI4AI is “to transform the state of agriculture by deploying emerging technologies in an inclusive and sustainable way.”
It seeks to evolve scalable frameworks for enabling the use of these technologies for constructive outcomes while underlining the challenges and unintended consequences they may bring.
While the program primarily focuses on technological innovation, it also acknowledges the importance of investing in low-tech interventions, developing novel and daring policies, increasing resource efficiency, fostering trust and transparency, collaborating across independent working groups, and achieving common goals.
The key objectives of AI4AI are:
To enhance digital and financial inclusivity among smallholder farmers.
To build trust and transparency through quality and traceability.
To protect the environment from unsustainable practices.
To establish sustainable farm incomes.

C4IR (Centre for the Fourth Industrial Revolution) India assisted in bringing the stakeholders together from November 2020 to February 2021 to set shared goals and foster innovation.
The potential effects of particular technology and methods to harness them for beneficial effects in India's agricultural system were discovered after four months of constant communication and collaboration.
The next portion of this report goes into depth about the main conclusions from this stage of the study.
The AI4AI program has also launched pilot projects in five districts of Telangana state, focused on priorities in the four defined thematic areas:
Intelligent Crop Planning and Sowing – “Sow the right crop in the right soil at the right time”.
Smart Farming – “maximize productivity, minimize input costs”.
Farmgate-to-Fork – “sell produce at the right time to the right buyer at the right price”.
Data-driven Agriculture – data governance and data regulation for smart decisions.
The Centre has created a steering committee to coordinate the working groups and their interactions with the wider program.
This multi-stakeholder advisory body has the following tasks:
Provide overall guidance, support, and direction to the working groups to help them operate effectively towards achieving their aims.
Coordinate activities across WGs to encourage synergies and convergence between them and to avoid duplication of effort.
Validate and approve the frameworks and action plans designed by the WGs in their respective areas.
Explore synergies between the AI4AI program and other national initiatives.
In partnership with C4IR India and the World Economic Forum, explore synergies and convergence with other projects addressing the UN Sustainable Development Goals (SDGs), to meet local challenges and opportunities, and to promote investments.
AI4AI impact objectives
The AI4AI initiative is aimed at demonstrating value to the main stakeholder of the agriculture sector, namely the farmer, through a multi-stakeholder approach.
The AI4AI impact objectives for phase 1 are defined as follows:
One goal: the economic development of farmers through a multi-stakeholder approach
10 streams of new value (e.g., seven use cases across three different themes)
100 farmer-producer organizations
1,000 villages
100,000 farmers
It is proposed that states can select a minimum of 10 new value streams to measure impact and validate frameworks out of the themes and use cases identified as game changers in this report, as listed below.
Designing an AI-driven macro-crop planning model for 10 integrated crop-planning units.
Providing pre-season crop/varietal advisories in project areas.
Smart FaaS (farming-as-a-service), an ET-based one-stop-shop for farmers’ needs throughout the Agri-cycle.
Creating e-SHCs (e-soil health cards) and making them accessible online to the target group of farmers.
Implementing smart crop insurance in the entire project area.
Demonstrating traceability of agricultural produce in respect of high-value Agri-produce.
Designing an AI-driven market intelligence system.
Establishing an AI-and IoT-based platform with farmer/value chain/buyer linkages, integrating quality and traceability.
Establishing a data marketplace platform for selected datasets, which could include data on weather, soil, inputs, and crops grown.
Creating an EFR (electronic farm record) for each farmer in the target group.
Some specific examples of AI in agriculture include:
Automated Farming Systems: AI-powered robots and drones can perform various tasks such as planting seeds, applying fertilizers and pesticides, and even harvesting crops. These automated farming systems not only reduce labor costs but also improve efficiency and accuracy in carrying out farming operations.
Crop Monitoring and Disease Detection: AI algorithms can analyze sensor data from satellites, drones, or IoT devices to monitor crop health and detect early signs of diseases.
Crop yield prediction: AI systems are used to analyze various factors such as weather conditions, soil quality, historical data, and crop characteristics to accurately predict crop yields. This helps farmers plan their planting and harvesting schedules, optimize resource allocation, and anticipate potential challenges or opportunities.
Smart irrigation: AI-powered systems use real-time data from sensors to determine the precise amount of water needed by crops. By analyzing factors such as weather conditions, soil moisture levels, and crop water requirements, these systems can optimize irrigation schedules and minimize water wastage.
Plant disease detection: AI algorithms can analyze images of plants taken by drones or smartphones to identify signs of disease or pest infestation. By quickly detecting these issues, farmers can take prompt action to mitigate the spread of diseases and protect their crops.
Automated harvesting: AI technology can be used to develop robotic systems that can autonomously harvest crops. These systems use computer vision and machine learning algorithms to identify and differentiate between ripe and unripe crops, enabling efficient and accurate harvesting.
Livestock monitoring: AI-powered sensors and tracking devices can be used to monitor the health and behavior of livestock. By collecting data on factors such as heart rate, temperature, activity levels, and feeding patterns, AI systems can detect signs of illness or distress in animals.
Source: AI4AI World Economic Forum, March 2021.
AI for Agri Innovation
- K H Akhil Srinivas
(KHAS)
Comments